In today’s rapidly evolving world, both technology and financial services are undergoing transformations that benefit consumers in unexpected ways. One of the most exciting developments is the integration of predictive analytics into the life insurance industry. This innovative approach is not only revolutionizing underwriting and risk management for insurers but also unveiling hidden life insurance benefits that many policyholders might never have discovered. In this comprehensive article, we’ll explore how predictive analytics works, how it’s reshaping life insurance, and why this could be the key to unlocking additional value in your policy.
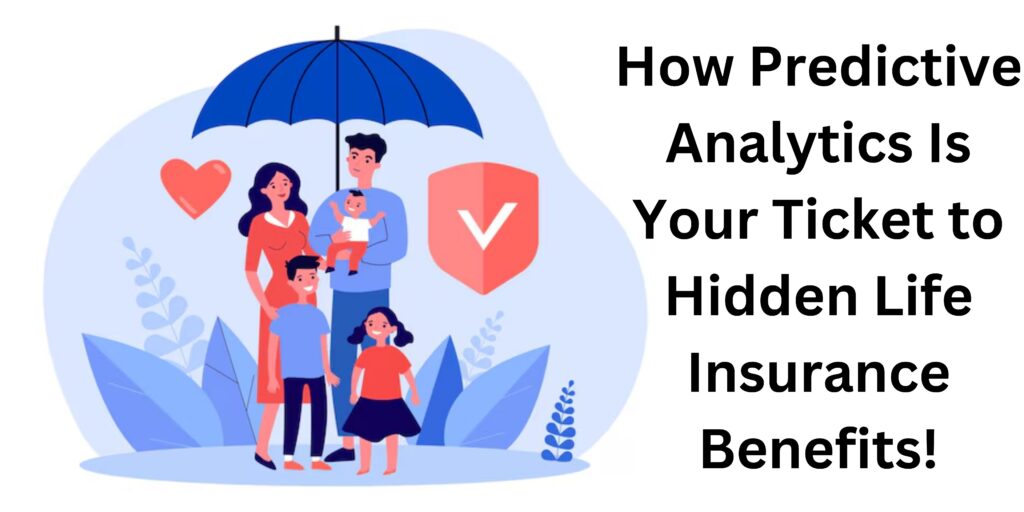
1. Understanding Life Insurance and Its Hidden Benefits
Life insurance is designed to provide financial protection to your loved ones when you’re no longer around. While most people understand that a life insurance policy offers a death benefit, there are numerous hidden advantages that extend beyond the basic coverage. These often include:
- Cash Value Accumulation: Many policies, such as whole life or universal life insurance, build cash value over time that can be accessed through policy loans or withdrawals.
- Accelerated Death Benefits: Some policies offer riders that allow policyholders to access a portion of the death benefit if diagnosed with a terminal illness.
- Customization through Riders: Additional benefits like critical illness coverage, disability riders, and waiver of premium riders can be attached to policies.
- Wellness Programs: Insurers are increasingly offering wellness programs that reward healthy behavior, often reducing premiums or increasing policy benefits.
- Tax Advantages: Life insurance policies can provide tax-deferred growth on the cash value component and tax-free death benefits in many circumstances.
These benefits are sometimes hidden in the fine print or underutilized because the average policyholder may not be aware of their full potential. With advancements in technology, predictive analytics is playing a crucial role in surfacing these advantages.
Read more:
2. What is Predictive Analytics?
Predictive analytics involves using historical data, statistical algorithms, and machine learning techniques to forecast future events. In simple terms, it’s the science of making predictions about future outcomes based on data patterns. Here’s how it works:
- Data Collection and Aggregation: Large volumes of data, from demographics and health records to lifestyle habits and even real-time wearable data, are collected.
- Data Analysis: Advanced algorithms process this data to identify trends, correlations, and potential risks.
- Forecasting Outcomes: By applying statistical models, predictive analytics forecasts future behaviors such as health risks, policy lapses, or claim likelihoods.
- Decision-Making: Insurers then use these predictions to make informed decisions about underwriting, pricing, and the customization of policies.
This approach is increasingly valuable in life insurance, where understanding individual risk profiles can lead to better, more personalized policies.
3. The Intersection of Predictive Analytics and Life Insurance
The marriage of predictive analytics and life insurance is a game changer. Traditionally, life insurance underwriting was based on generalized tables and limited data. Today, insurers leverage vast amounts of information to assess individual risk more accurately. This evolution offers several advantages:
Enhanced Underwriting
By incorporating detailed health data and lifestyle information, insurers can:
- Assess Risk with Greater Precision: Detailed risk models lead to more accurate pricing.
- Identify Healthy Behaviors: Rewarding healthy lifestyle choices with lower premiums.
- Minimize Uncertainty: Improved data leads to fewer surprises when claims are made.
Personalized Insurance Policies
Predictive analytics allows insurers to move away from one-size-fits-all policies. With the power of data, they can:
- Tailor Policies to Individual Needs: Design products that meet specific financial and health profiles.
- Offer Customized Riders: Identify which additional coverages may benefit the policyholder.
- Optimize Cash Value Accumulation: Suggest policy modifications to maximize benefits over time.
Operational Efficiency
From streamlining claims processing to proactive customer engagement, predictive analytics helps insurers:
- Detect Fraud: Identify anomalies in claims that may indicate fraud.
- Enhance Customer Service: Anticipate customer needs and provide timely solutions.
- Improve Retention Rates: By offering tailored services and benefits, policyholders are more likely to stay with the insurer.
4. How Predictive Analytics Uncovers Hidden Life Insurance Benefits
Predictive analytics doesn’t just help insurers manage risk—it actively uncovers hidden benefits that add value for policyholders. Here’s how:
4.1 Identifying Underutilized Policy Riders
Many life insurance policies come with optional riders that offer additional coverage. However, not every policyholder is aware of these benefits. Predictive models analyze customer data to identify:
- Health Trends: For instance, if an individual’s health data indicates a higher risk of certain illnesses, insurers might suggest adding a critical illness rider.
- Lifestyle Factors: Data from wearable devices or regular health assessments can reveal when a wellness rider or disability rider might be beneficial.
- Behavioral Patterns: Predictive analytics can spot trends in lifestyle changes, prompting recommendations for policy adjustments that enhance coverage.
4.2 Optimizing Cash Value and Investment Components
For policies that build cash value over time, predictive analytics can help in several ways:
- Maximizing Growth: By analyzing market trends and individual risk factors, analytics can suggest strategies for better cash value accumulation.
- Strategic Policy Loans: Predictive tools can forecast the optimal time for taking policy loans, ensuring minimal impact on the death benefit while providing needed liquidity.
- Investment Allocation: Some policies offer investment options; predictive models can advise on adjusting these allocations to suit the policyholder’s long-term goals.
4.3 Enhancing Wellness Programs
Many insurers are integrating wellness programs that promote healthy living by offering premium discounts or additional benefits. Predictive analytics plays a crucial role here:
- Tracking Health Metrics: Continuous monitoring through wearables provides data that can be analyzed for trends.
- Rewarding Healthy Behaviors: Insurers can tailor rewards based on actual behavior rather than static data.
- Dynamic Adjustments: Predictive models can dynamically adjust benefits as the policyholder’s health data evolves.
4.4 Customized Risk Management
Traditional underwriting might label a policyholder with a high risk based solely on broad statistical data. However, predictive analytics allows for:
- Personalized Risk Assessment: Detailed analysis helps in understanding the unique risk profile of each individual.
- Proactive Mitigation Strategies: By predicting potential future health issues, insurers can suggest lifestyle changes or policy modifications to mitigate risk.
- Flexible Coverage Options: Policyholders can receive recommendations for additional coverages that better align with their evolving life circumstances.
4.5 Real-World Example: A Hypothetical Case Study
Imagine a 45-year-old professional who has a whole life policy with a modest cash value component. Over the years, the insurer has collected data on the individual’s health, lifestyle, and wellness program participation. With predictive analytics, the insurer might discover that:
- The policyholder’s regular engagement in physical activity and participation in health check-ups significantly reduce their risk profile.
- There is an opportunity to optimize the cash value accumulation through minor policy adjustments.
- The individual could benefit from an accelerated death benefit rider in the event of a serious illness, which had not been initially highlighted.
Based on these insights, the insurer could proactively reach out with a personalized offer to modify the policy, ensuring that the policyholder gains access to hidden benefits that enhance both their current well-being and long-term financial security.
5. Implementing Predictive Analytics in Life Insurance: The Process
For insurers looking to harness the power of predictive analytics, the journey involves several key steps:
5.1 Data Collection
The first step is to gather diverse data sources:
- Internal Data: Historical claims, policyholder profiles, underwriting records.
- External Data: Market trends, socioeconomic data, public health records.
- Real-Time Data: Wearable technology, mobile apps, customer interactions.
5.2 Data Cleaning and Integration
Before analysis, data must be cleaned and integrated:
- Standardization: Ensuring that data from various sources is compatible.
- Error Correction: Identifying and rectifying anomalies or incomplete data.
- Integration: Combining disparate data sets into a cohesive format for analysis.
5.3 Feature Engineering
Feature engineering involves identifying and selecting the most relevant variables that will influence predictions:
- Health Indicators: Metrics like blood pressure, cholesterol levels, and physical activity.
- Behavioral Patterns: Lifestyle habits such as diet, exercise frequency, and sleep patterns.
- Demographic Factors: Age, gender, occupation, and family medical history.
5.4 Model Building
Using statistical models and machine learning algorithms, insurers can build predictive models:
- Risk Models: To predict the likelihood of claims or health deterioration.
- Pricing Models: To adjust premiums based on personalized risk profiles.
- Benefit Optimization Models: To identify the best ways to maximize policy benefits.
5.5 Evaluation and Continuous Improvement
After deployment, models must be continuously evaluated:
- Performance Metrics: Assessing model accuracy, precision, and recall.
- Feedback Loops: Incorporating new data to refine predictions.
- Regulatory Compliance: Ensuring that models adhere to legal and ethical standards.
By following this structured process, insurers can deploy predictive analytics effectively, turning raw data into actionable insights that benefit both the company and its policyholders.
6. Benefits for Policyholders
The incorporation of predictive analytics into life insurance brings significant advantages to policyholders. Here’s a closer look at these benefits:
6.1 Personalized Policy Recommendations
Predictive analytics allows insurers to:
- Tailor Coverage: Policies are customized based on an individual’s unique health and lifestyle profile.
- Optimized Pricing: Enjoy lower premiums if healthy behaviors and lower risks are identified.
- Dynamic Adjustments: Policies evolve with changing circumstances, ensuring continuous alignment with the policyholder’s needs.
6.2 Improved Transparency and Trust
When insurers use data-driven methods:
- Clear Communication: Policyholders receive detailed explanations of how premiums and benefits are determined.
- Proactive Service: Early alerts on potential benefits or necessary policy changes enhance the customer experience.
- Enhanced Trust: Data-backed decisions build confidence in the insurer’s ability to manage risk responsibly.
6.3 Maximizing Hidden Benefits
Thanks to predictive analytics, policyholders can unlock:
- Enhanced Riders: Customized suggestions for riders that fit their lifestyle and risk profile.
- Optimized Cash Value: Strategic guidance on how to maximize the growth and utility of accumulated cash value.
- Wellness Incentives: Access to tailored wellness programs that not only improve health but also reward policy adherence with better benefits.
6.4 Financial Security and Flexibility
With predictive analytics:
- Proactive Risk Management: Early detection of potential issues means policyholders can adjust behaviors or modify policies before problems arise.
- Informed Decision-Making: Transparent, data-driven insights help customers make smarter financial decisions about their coverage.
- Long-Term Value: By continually aligning policies with real-time data, policyholders can secure benefits that adapt to their changing life circumstances.
7. Challenges and Considerations
While the benefits of predictive analytics in life insurance are considerable, there are also important challenges to address:
7.1 Data Privacy and Security
- Sensitive Information: Life insurance involves highly personal data. Ensuring that this data is protected against breaches is paramount.
- Regulatory Compliance: Adhering to data protection regulations such as GDPR or HIPAA is critical for maintaining consumer trust.
7.2 Accuracy of Predictions
- Model Limitations: Even sophisticated algorithms can sometimes produce inaccurate predictions due to incomplete or biased data.
- Continuous Monitoring: Regular model updates are necessary to maintain accuracy as new data emerges and conditions change.
7.3 Bias and Fairness
- Algorithmic Bias: There is a risk that models might inadvertently incorporate biases present in historical data.
- Ethical Considerations: Insurers must ensure that predictive analytics does not lead to unfair treatment of certain groups.
7.4 Transparency in Decision-Making
- Explainability: Complex algorithms can sometimes operate as “black boxes.” Policyholders deserve clear explanations of how decisions are made.
- Consumer Trust: Transparency is key to maintaining trust, especially when decisions have significant financial implications.
8. Future Trends in Predictive Analytics and Life Insurance
As technology continues to evolve, several trends are set to further integrate predictive analytics into the life insurance landscape:
8.1 Integration of IoT and Wearable Technology
- Real-Time Health Monitoring: Devices that track daily activity, sleep patterns, and vital signs will provide even richer data.
- Immediate Adjustments: Insurers may soon offer dynamic premium adjustments based on live health data, creating a more responsive policy framework.
8.2 Artificial Intelligence and Deep Learning
- Enhanced Modeling: AI will enable even more sophisticated risk assessment and benefit optimization models.
- Predictive Precision: Deep learning algorithms can uncover patterns in data that traditional models might miss, leading to more personalized recommendations.
8.3 Increased Customization and Flexibility
- On-Demand Coverage: Future policies might become more flexible, allowing adjustments in coverage or benefits in response to life changes.
- Customer Empowerment: With clearer data insights, customers will be better informed to make decisions that align with their lifestyle and financial goals.
8.4 Evolving Regulatory Environment
- Adaptive Regulations: As technology advances, regulators are likely to update guidelines to ensure consumer protection while fostering innovation.
- Industry Collaboration: Greater collaboration between insurers, technology providers, and regulators will drive ethical and effective use of predictive analytics.
9. FAQs
A: Predictive analytics uses historical and real-time data along with statistical algorithms and machine learning techniques to forecast future outcomes. In life insurance, it helps assess risk, personalize policies, optimize benefits, and uncover hidden advantages that traditional models may miss.
A: By analyzing data on your health, lifestyle, and even wearable device metrics, predictive models can identify opportunities such as underutilized policy riders, optimal times to access cash values, and personalized wellness incentives that enhance your policy’s overall value.
A: Yes, privacy is a key consideration. Insurers must comply with data protection regulations and use robust security measures to ensure that your personal information is handled safely and ethically.
A: Look for transparency in how your data is used, clear communication about how your risk and benefits are calculated, a strong track record of data security, and evidence of continuous improvement in their predictive models.
A: Absolutely. By accurately assessing your personal risk profile and rewarding healthy behaviors, insurers can offer lower premiums and tailor policy benefits to better meet your needs.
10. Conclusion
Predictive analytics is transforming the life insurance industry by making it more data-driven, personalized, and customer-centric. The traditional model of life insurance is evolving—no longer limited to static policies and generic coverage. Today, insurers are using sophisticated data analysis techniques to identify hidden benefits that many policyholders are unaware of.
From optimizing cash value accumulation to unlocking underutilized riders and rewarding healthy behaviors, predictive analytics empowers you to extract maximum value from your life insurance policy. While challenges such as data privacy, model accuracy, and algorithmic bias remain, ongoing advancements in technology and stricter regulatory frameworks are paving the way for a more transparent and equitable system.
By understanding how predictive analytics works and its impact on life insurance, you can take a proactive approach to managing your financial security. Whether you’re considering a new policy or reevaluating an existing one, it’s worth exploring how your insurer leverages data-driven insights to enhance your coverage and reveal hidden benefits.